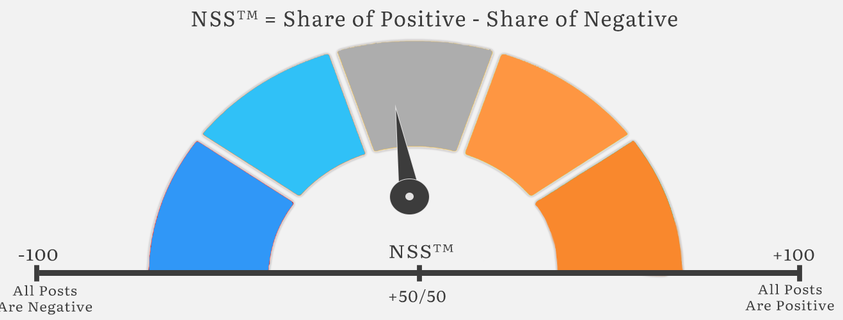
The Complete Story of DMR's NSS™ Score and Its Strategic Imperative
As businesses seek to understand their standing in the digital conversation, DMR's Net Sentiment Score (NSS™) emerges as a pivotal metric. ...
Probably the best next generation market research blog on the web